[Accepted by KBS] We propose a novel solution for solving the representation bottleneck. By theoratically provements, we verify the existence of the problem and successfully increase the representation ability of the Transformer model. Experiments are done with language modeling/GLUE tasks on Transformer/BERT models.
Authors
Zhong Zhang; Nian Shao; Chongming Gao; Rui Miao; Qinli Yang; Junming Shao
Abstract
The Transformer-based models have achieved significant advances in language modeling, while the multi-head attention mechanism in Transformers plays an indispensable part in their success. However, the too-small head size caused by the multi-head mechanism will lead to one problem called the low-rank bottleneck, which means that the rank of the attention weight matrix is too small to represent any desired attention. Naively increasing the head size is insufficient to solve the problem because it leads to severe parameter explosion and overfitting. To tackle this problem, we propose a mix-head attention (Mixhead) which mixes multiple attention heads by learnable mixing weights to improve the expressive power of the model. In contrast, Mixhead achieves a higher rank of the attention weight matrix while introducing a negligible number of parameters. Furthermore, Mixhead is quite general and can be easily adopted to most multi-head attention based models. We conduct extensive experiments including language modeling, machine translation, and finetuning BERT to demonstrate the effectiveness of our method.
URL
https://www.sciencedirect.com/science/article/pii/S0950705121011503
Code
The code for this article is not published
Main Results
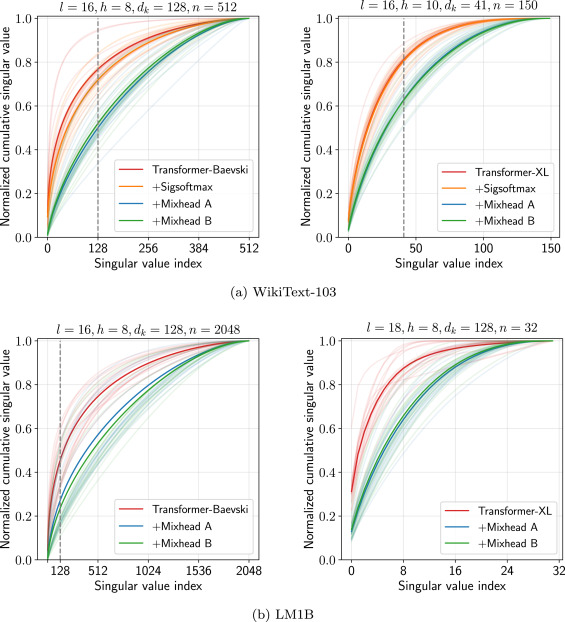