[Accepcted by ICASSP 2024] We propose a novel online EEND model for speaker diarization task, and obtain SOTA performances on the synthetic/CALLHOME datasets.
Authors
Di Liang; Nian Shao; Xiaofei Li
Abstract
This work proposes a frame-wise online/streaming end-to-end neural diarization (FS-EEND) method in a frame-in-frame-out fashion. To frame-wisely detect a flexible number of speakers and extract/ update their corresponding attractors, we propose to leverage a causal speaker embedding encoder and an online non-autoregressive self-attention-based attractor decoder. A look-ahead mechanism is adopted to allow leveraging some future frames for effectively detecting new speakers in real time and adaptively updating speaker attractors. The proposed method processes the audio stream frame by frame, and has a low inference latency caused by the look-ahead frames. Experiments show that, compared with the recently proposed block-wise online methods, our method FS-EEND achieves state-of-the-art diarization results, with a low inference latency and computational cost.
URL
https://arxiv.org/abs/2309.13916
Code
Network Architecture
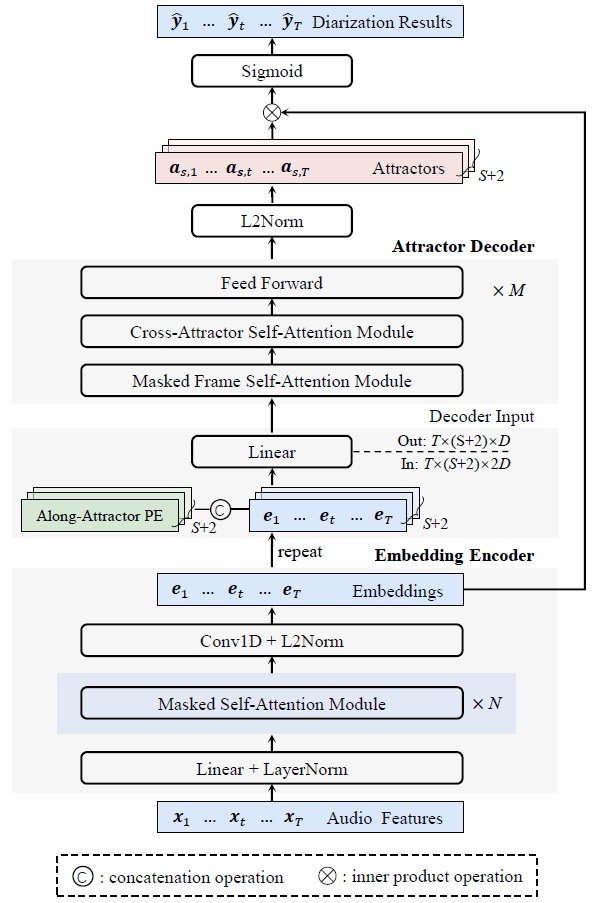